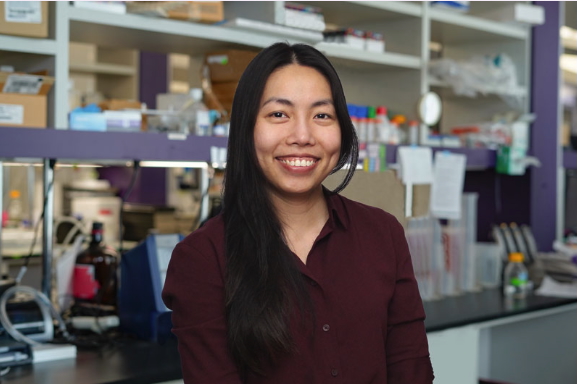
Omics research fields, such as genomics (the study of an organism's entire DNA), analyze the complete set of a specific type of biological molecule within an organism. Other forms of omics include proteomics, which looks at proteins, and transcriptomics, which focuses on all RNA molecules in an organism.
Omics are an essential part of ALS research. Learning more about the role DNA, RNA, proteins, and other factors might play in different cases of ALS could reveal important insights about causes, risk factors, or even potential avenues of treatment for the disease. That’s why the collection of a variety of omics data is a key pillar of the ALS Therapy Development Institute’s (ALS TDI) ALS Research Collaborative (ARC) Study, the longest-running natural history study in ALS.
ARC researchers have collected a vast number of biological samples from people with ALS and asymptomatic carriers of ALS-related genetic mutations since the study’s launch in 2014. Analyzing the omics data available in these samples has the potential to unlock important insights about ALS, but it is a serious undertaking. Every sample could include hundreds of thousands or even millions of datapoints—different genes, proteins, or RNA molecules that could tell us something about that person’s disease.
Advanced data science techniques are essential to digging through these data and finding meaningful discoveries. Recently, Dr. Gwen Huynh, Ph.D., joined ALS TDI’s team as a Senior Data Scientist focusing specifically on omics research. She recently sat down with us to talk about the challenges and opportunities presented by these large-scale omics datasets, and what it’s been like joining ALS TDI’s field-leading science team.
ALS TDI: First off, can you introduce yourself and say a little bit about what you do at ALS TDI?
Gwen Huynh: I’m Gwen Huynh I recently started at ALS TDI as a Senior Data Scientist. I cover the omics part of things. So, genomics, proteomics, transcriptomics. Omics, basically, are like a collection of everything we can find in a biological sample.
Genomics is a collection of all the genes. Proteomics is a collection of all the proteins that we can find within a sample. The sample can be a patient's blood, or a cell culture, and so on and so forth.
Can you say a little bit about what you’re working on and how it fits into ALS TDI’s larger research picture?
There's a couple of big questions that we want to ask in terms of how we can advance ALS research and help ALS patients. One is trying to find a blood biomarker, or a couple of blood biomarkers, that could help us predict the progression of the disease. With blood biomarkers, someone with ALS could come in and give blood samples, and then based on the blood biomarkers, we could predict how your disease is going to change over time.
For that, we're using proteomics. We're taking snapshots of the proteins in a patient’s blood sample and then trying to correlate that with the data that we have from patients who have given blood previously.
The other part that I'm handling is full genome sequencing. What we’re doing is trying to identify risk factors for ALS. We're trying to look at the genomics of the patients that we have samples for and trying to correlate it with other external data to see if we can draw a correlation or causation between what we call risk factors and disease progression.
Can you say a little more about where the data you’re working with comes from?
The data comes from the ALS Research Collaborative (ARC) study, which has been collecting data about ALS for more than 10 years. We have a cohort of about 1000 people who have submitted data. About 600 of those have undergone whole genome sequencing. We also have longitudinal data from people who have given blood every couple of months over a period of time so that, again, we can look at what a blood marker might look like at month zero versus month three. If you're progressing, we can look at what kind of factors, such as what kind of proteins in your blood, might correlate with your progression.
You’re relatively new at ALS TDI. What was your first impression of the data you’re working with here like?
I was very excited when I joined, and I'm still very excited. The quality of the data that we have are top-notch. It’s very well annotated, which is very important if you want to apply any of these machine learning algorithms. The first part of the process is that you have to label the data correctly. It has to be right, and it has to be meticulous. And I think ALS TDI did a very good job of that.
What are some of the challenges of looking at these kinds of datasets and asking the questions that you’re asking?
I think the challenge is that ALS is a very complex disease. There's not one factor that causes it. We're still figuring out: “If there is a combination of factors, what are they? Are they applicable from patient to patient?” Because if every patient is different, then it's very hard to predict for a bigger population.
What are some of the specific projects you’re currently working on since you’ve started at ALS TDI?
Currently, I'm working directly with Answer ALS as part of a collaboration between ALS TDI, Answer ALS, and Microsoft to create a portal for genomics data so that it is accessible to other ALS researchers. That's my current project right now.
I'm also working with [ALS TDI Director of Clinical Operations] Alan Premasiri and [AS TDI Senior Director of Cell Biology] Dr. Kyle Denton on specific projects. Alan has been helping me get to know the clinical data from the ARC Study so that I can understand how the data are annotated.
I’m working with Kyle on studying induced Pluripotent Stem Cell (iPSC) models of ALS. I'm actually very excited, once I get this collaborative project with Answer ALS done, to move on to Kyle’s project of analyzing the transcriptomes of iPSC-derived neurons to understand more of the difference between normal neurons and neurons of patients who are affected by ALS. I think that's very exciting.
To that point, what’s it like to join an organization with so many different aspects of preclinical research—like cell biology and translational research—happening under one roof?
I think it shortens your discovery time. I really treasure the dynamic that we have where you can just go talk to Kyle about cell biology or talk to Alan about clinical data, and they’re experts in what they do. They know so much about the research and the data itself.
I imagine it might be a bit harder if you were to outsource to a contract research organization that’s doing iPSCs. You’d probably have to schedule a meeting to talk about the results. So, because all this research is happening under one roof at ALS TDI, it’s more streamlined.
You’ve talked about some of the projects you’re working on as you start your time at ALS TDI. What are some other things you’re excited to investigate in the future?
My background is in cell biology, and my hope is that I can eventually use some of these exciting new AI tools and apply them to our omics data. Right now, there’s a lot of AI software being used for things like writing or marketing. AI in omics is really in its infancy, but a lot of people are working on it.
I’m really excited because I feel like we’re at the point where we have good data that reaches the amounts that are needed to train these AI tools. The data are very good in terms of how they’re annotated and labeled. And the AI tools that are out there have also gotten very good in parallel. So, it’s an exciting opportunity to apply these very good new tools with these very good data.
To learn more about ALS TDI and our research to end ALS, click here.
What to Do Next: